The Future of Intelligent Grid: Integrating Smart Technologies in Energy Management Systems
1. Introduction
The global energy landscape is undergoing a transformative shift, driven by the increasing adoption of renewable energy, the decentralisation of power generation, and the growing demand for energy efficiency. The integration of smart power electronics, Artificial Intelligence (AI), Machine Learning (ML), and Industrial Internet of Things (IIoT) has emerged as a game-changer for modernising grid infrastructure, particularly in the management and optimisation of intelligent grids. This integrated system enables intelligent grid control, predictive maintenance, and optimised energy management for commercial and industrial customers, as well as end-users. By leveraging these technologies, utilities and businesses can achieve higher reliability, efficiency, and sustainability in their energy systems.
2. Intelligent Grid Control: The Backbone of Modern Energy Systems
The integration of smart power electronics, AI, ML, and industrial IoT enhances real-time monitoring and control of the grid. This ensures that the grid operates efficiently and reliably, even under varying conditions.
2.1 The Role of Smart Power Electronics
Smart power electronics form the foundation of intelligent grid control. Devices such as solid-state transformers (SSTs),advanced inverters, and flexible AC transmission systems (FACTS) enable precise control of voltage, current, and frequency in real-time.
These devices are essential for integrating renewable energy sources (e.g., solar, wind, geothermal) and distributed energy resources into the grid, ensuring stability and efficiency. For example, advanced inverters with Maximum Power Point Tracking (MPPT) algorithms optimise the output of solar panels and wind turbines in renewable integration, even under varying weather conditions. The FACTS devices, such as Static VAR Compensators (SVCs), can be deployed to dynamically regulate reactive power for preventing voltage fluctuations and blackouts and maintaining grid stability.
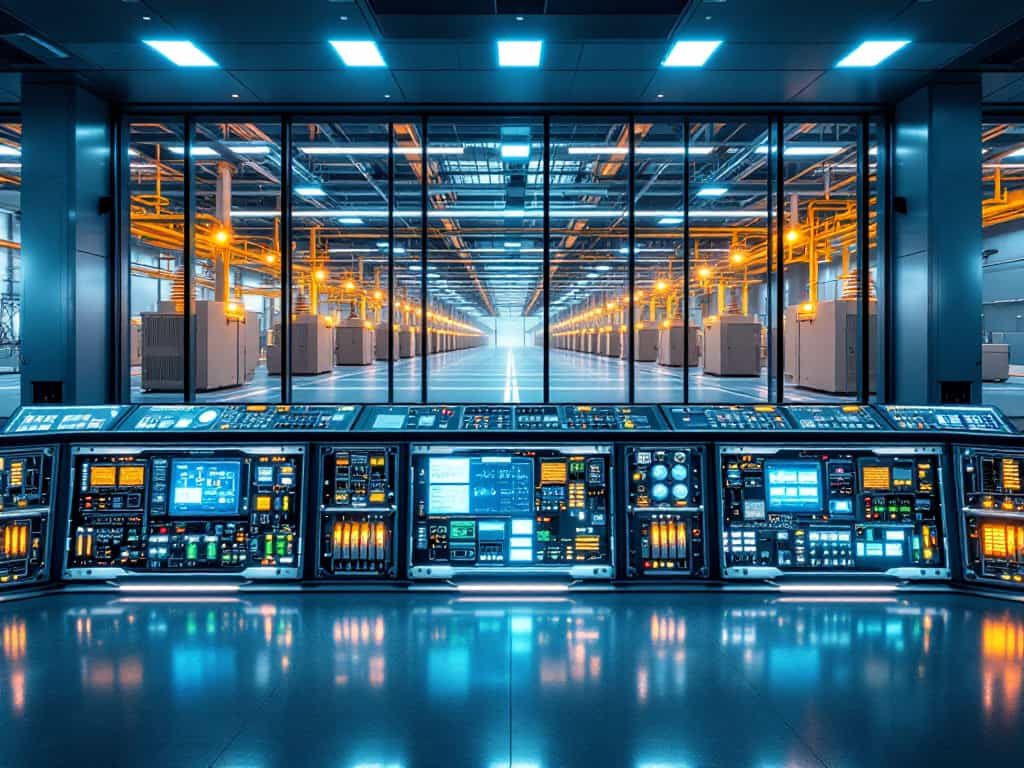
2.2 AI and ML for Real-Time Decision Making
AI and ML algorithms enhance the capabilities of smart power electronics by enabling real-time decision-making and adaptive control. These technologies analyse vast amounts of data from sensors, smart meters, and historical grid performance to optimise grid operations, energy distribution and detect anomalies in real-time.
- Demand Response: AI-based systems predict peak demand periods and automatically adjust energy distribution to avoid overloads. For example, during a heatwave, the system can prioritise cooling for critical facilities while reducing supply to non-essential loads.
- Fault Detection and Isolation: ML models trained on historical fault data can identify anomalies (e.g., voltage sags, line faults) and isolate affected sections within milliseconds, minimising downtime.
2.3 IIoT: Enabling Connectivity and Automation
Industrial IoT refers to the network of physical devices, vehicles, home appliances, and other items embedded with electronics, software, sensors, actuators, and connectivity which enables these objects to connect and exchange data. In the energy sector, IIoT devices, such as smart sensors and edge computing units, collect real-time data on grid parameters, equipment performance, and environmental factors, and provide the connectivity needed for real-time monitoring and control. These devices collect data from grid assets (e.g., transformers, substations) and transmit it to centralised or decentralised control systems.
IIoT enables decentralised control architectures, where multiple nodes (e.g., microgrids) operate autonomously while coordinating with the main grid. This approach enhances resilience and reduces the risk of cascading failures. By processing data locally at the edge, IIoT devices reduce latency and enable faster response times. For instance, an edge device can detect a transformer overheating and trigger a cooling system without waiting for a central command.
3 Predictive Maintenance: Reducing Downtime and Costs
3.1 The Need for Predictive Maintenance
Traditional maintenance practices, such as time-based or reactive maintenance, are no longer sufficient for modern grids. These methods are either too costly (e.g., replacing components prematurely) or too risky (e.g., waiting for equipment to fail). Predictive maintenance, powered by AI, ML, and IIoT, offers a more efficient and cost-effective alternative.
3.2 AI and ML for Asset Health Monitoring
AI and ML algorithms analyse data from IIoT sensors to predict equipment failures before they occur. These algorithms consider factors such as temperature, vibration, and load history to assess the health of grid assets. For example, by analysing dissolved gas levels in transformer oil, ML models in a Transformer Health Monitoring system can predict insulation degradation and recommend maintenance actions. To predict cable fault, AI algorithms detect early signs of cable wear (e.g., partial discharges) and alert operators to replace the cable before a failure occurs.
3.3 IIoT for Condition-Based Monitoring
IIoT sensors provide real-time data on the condition of grid assets, enabling condition-based maintenance. For example, the vibration sensors can detect imbalances in rotating machinery (e.g., turbines) and trigger maintenance alerts. By using thermal imaging cameras, the operator can monitor hotspots in electrical panels and switchgear, preventing overheating-related failures.
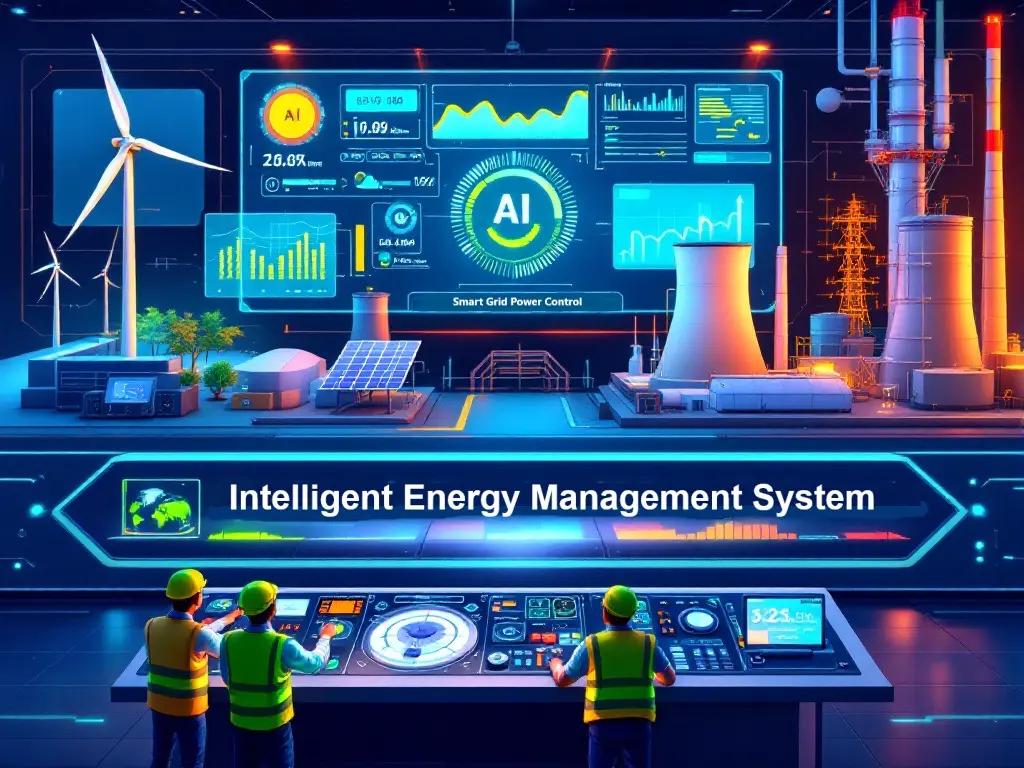
3.4 Benefits of Predictive Maintenance
By adopting predictive maintenance techniques, it can reduced downtime to address issues before they escalate for minimises unplanned outages due to ageing or malfunctioning equipment. It can also optimise maintenance schedules to reduce unnecessary replacements and labour costs. In addition, it can extend asset lifespan to ensures that equipment operates within optimal conditions for prolonging its useful life.
4. Energy Optimisation: Empowering Commercial and Industrial Customers
4.1 Smart Energy Management Systems
AI and ML-powered energy management systems (EMS) enable commercial and industrial customers to optimize their energy consumption and reduce costs. These systems analyse energy usage patterns, weather forecasts, and tariff structures to recommend optimal energy strategies. AI algorithms shift energy-intensive processes to off-peak hours, taking advantage of lower tariffs. In integrated renewable energy power system, ML models predict solar and wind generation, enabling businesses to maximise the use of on-site renewables.
4.2 IIoT for Real-Time Energy Monitoring
IIoT devices provide real-time visibility into energy consumption at the device level. For example, smart meters can track energy usage in real-time and provide granular data for analysis. In energy dashboards, it displays energy consumption trends and anomalies, helping businesses identify inefficiencies.
4.3 Demand-Side Management
AI and IIoT enable demand-side management (DSM) strategies, where customers adjust their energy usage in response to grid conditions. For example, during periods of high demand, utilities can offer incentives for customers to reduce consumption. Regulated by automated load control technique, smart thermostats and lighting systems automatically adjust settings to reduce energy usage during peak periods.
4.4 Improved Energy Efficiency
The integrated system enables optimized energy management, reducing waste and improving energy efficiency. For example, AI algorithms can analyse data from smart meters and sensors to identify opportunities for energy savings, such as reducing peak demand or optimizing energy use in buildings. This not only reduces energy costs but also contributes to sustainability goals.
4.5 Enhanced Customer Experience
The integrated system provides commercial and industrial customers, as well as end-users, with valuable insights into their energy consumption. This enables them to make informed decisions about their energy use, reducing costs and improving sustainability. For example, AI-powered smart meters can provide customers with real-time data on their energy consumption, enabling them to identify opportunities for energy savings.
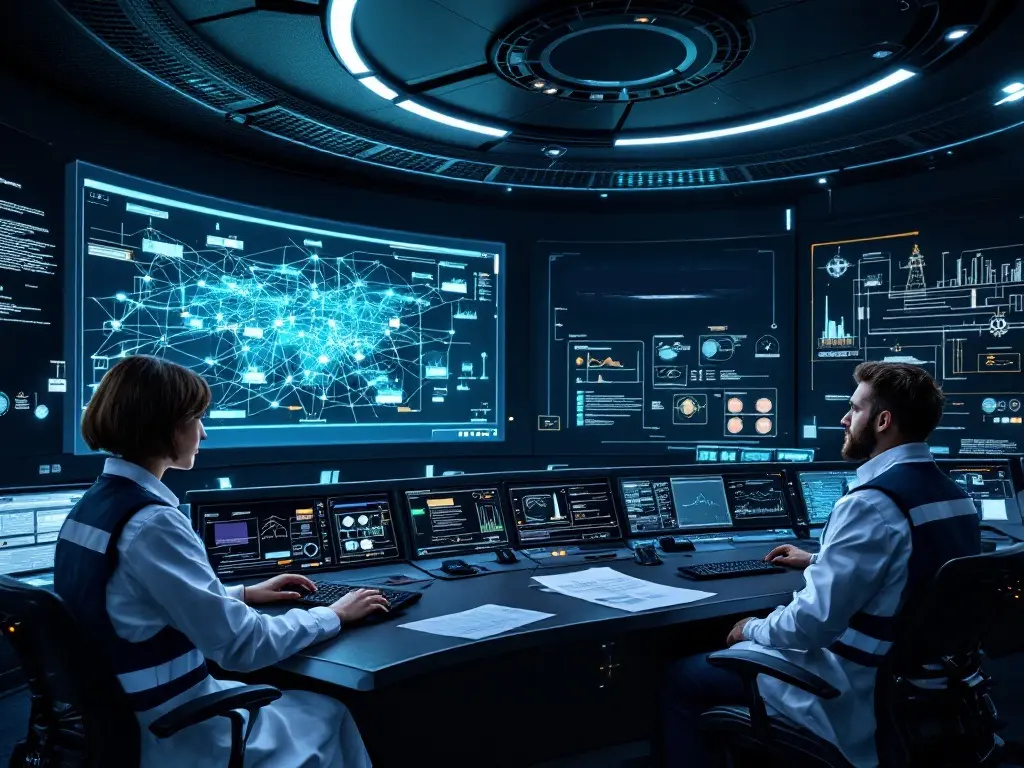
5. Challenges and Future Trends
5.1 Challenges
5.2 Future Trends
The integration of smart power electronics, AI, ML, and industrial IoT is still in its early stages, and there are many opportunities for further development and innovation. Some future directions include:
6. Conclusion
The integration of smart power electronics, AI, ML, and IIoT is transforming the way we control, maintain, and optimise energy management systems. These technologies is revolutionising grid operations on three key areas: intelligent grid control, predictive maintenance, and energy optimisation. For commercial and industrial customers, these technologies offer unprecedented opportunities to reduce costs, improve reliability, and enhance sustainability and energy consumption prediction. As the energy landscape continues to evolve, the adoption of these technologies will be critical for building a resilient, efficient, and intelligent grid. By addressing the challenges and embracing future trends, utilities and businesses can unlock the full potential of this integrated system, paving the way for a smarter and more sustainable energy future. The future of energy is intelligent, and the integration of smart power electronics, AI, ML, and industrial IoT is leading the way.
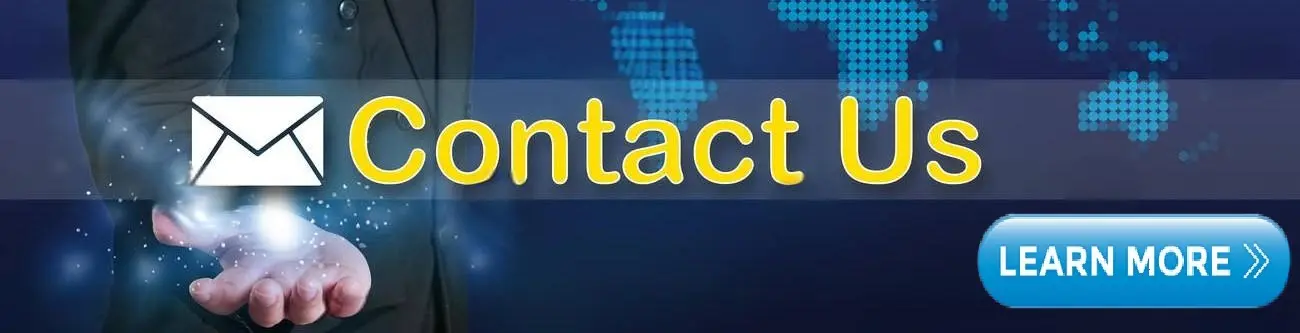
Back to the list